April 3, 2024
Editors' notes
This article has been reviewed according to Science X's editorial process and policies. Editors have highlighted the following attributes while ensuring the content's credibility:
fact-checked
peer-reviewed publication
proofread
Research team develops reconfigurable photonic computing architecture for lifelong learning
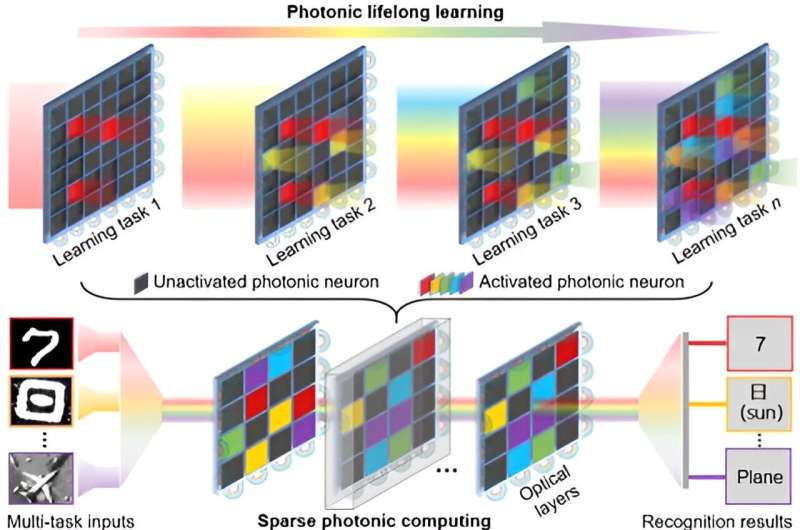
Artificial intelligence (AI) tasks have become increasingly abundant and complex, fueled by large-scale datasets. With the plateau of Moore's law and end of Dennard scaling, energy consumption becomes a major barrier to more widespread applications of today's heavy electronic deep neural models, especially in terminal/edge systems.
The community is seeking next-generation computing modalities to break through the physical constraints of electronics-based implementations of artificial neural networks (ANNs).
Photonic computing has been a promising avenue for overcoming the inherent limitations of electronics and improving energy efficiency, processing speed and computational throughput by orders of magnitude.
Such extraordinary properties have been exploited to construct application-specific optical architectures for solving fundamental mathematical and signal processing problems with performances far beyond those of existing electronic processors.
Unfortunately, existing ONNs suffer "catastrophic forgetting" are still struggling with simple onefold tasks. The main reason is that they inherit the widespread problem of conventional computing systems, which are prone to training new models that interfere with formerly learned knowledge, rapidly forgetting the expertise gained from previously learned tasks when trained on something new.
Such an approach fails to fully exploit the intrinsic properties in sparsity and parallelism of wave optics for photonic computing, which ultimately results in poor network capacity and scalability for multi-task learning.
In a recent paper published in Light: Science & Applications, a team of scientists, led by Professor Lu Fang from Sigma Laboratory, Department of Electronic Engineering, Tsinghua University, Beijing, China, and co-workers have developed L2ONN, a reconfigurable photonic computing architecture for lifelong learning.
The unique characteristics of light, spatial sparsity and multi-spectrum parallelism have been developed in photonic computing architecture for the first time, endowing ONNs with lifelong learning capability. Unlike existing ONNs that try to imitate ANN structures, the photonic lifelong learning of L2ONN is initially designed following the physical nature of light–matter interaction, to fully explore the functional and performance potentials of wave optics in photonic computing.
Benefiting from the proposed lifelong-learning optical computing architecture, experimental evaluations on free-space and on-chip architectures demonstrate that L2ONN shows its extraordinary learning capability on challenging tens-of-tasks, such as vision classification, voice recognition and medical diagnosis, supporting various new environments.
L2ONN achieves up to 14 times larger capacity than existing optical neural networks, with an order of magnitude higher energy efficiency than the representative electronic artificial neural networks.
"Humans possess the unique ability to incrementally absorb, learn and memorize knowledge. In particular, neurons and synapses perform work only when there are tasks to deal with, in which two important mechanisms participate: sparse neuron connectivity and parallelly task-driven neurocognition, together contribute to a lifelong memory consolidation and retrieval.
"Accordingly, in ONNs, these characteristic features can be naturally promoted from biological neurons to photonic neurons based on the intrinsic sparsity and parallelism properties of optical operators.
"An optical architecture imitating the structure and function of human brains demonstrates its potential to alleviate the aforementioned issues, which shows more advantages than electronic approaches in constructing a viable lifelong learning computing system.
"We have demonstrated the photonic lifelong learning provides a turnkey solution for large-scale real-life AI applications with unprecedented scalability and versatility. We anticipate that the proposed neuromorphic architecture will accelerate the development of more powerful photonic computing as critical support for modern advanced machine intelligence and towards beginning a new era of AI," stated the scientists.
More information: Yuan Cheng et al, Photonic neuromorphic architecture for tens-of-task lifelong learning, Light: Science & Applications (2024). DOI: 10.1038/s41377-024-01395-4
Journal information: Light: Science & Applications Provided by TranSpread Citation: Research team develops reconfigurable photonic computing architecture for lifelong learning (2024, April 3) retrieved 3 April 2024 from https://techxplore.com/news/2024-04-team-reconfigurable-photonic-architecture-lifelong.html This document is subject to copyright. Apart from any fair dealing for the purpose of private study or research, no part may be reproduced without the written permission. The content is provided for information purposes only.
Explore further
Multi-synaptic photonic spiking neural networks based on a DFB-SA chip 16 shares
Feedback to editors