November 20, 2024
Editors' notes
This article has been reviewed according to Science X's editorial process and policies. Editors have highlighted the following attributes while ensuring the content's credibility:
fact-checked
trusted source
proofread
In the 'Wild West' of AI chatbots, subtle biases related to race and caste often go unchecked
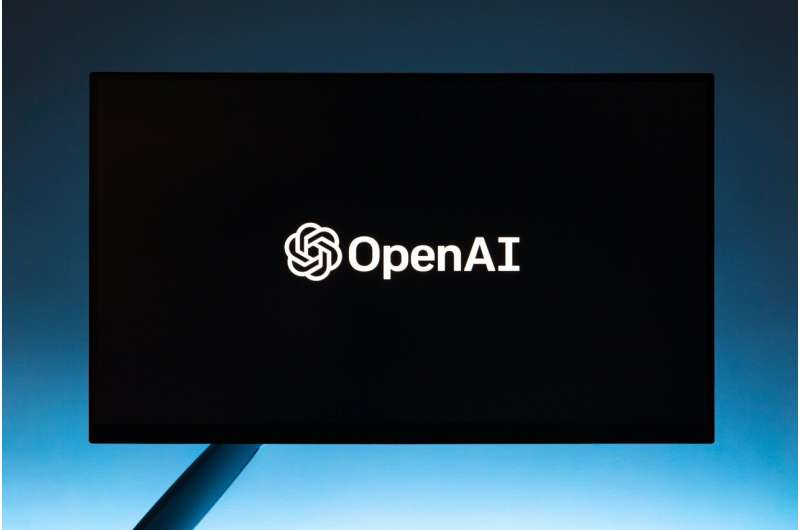
Recently, LinkedIn announced its Hiring Assistant, an artificial intelligence "agent" that performs the most repetitious parts of recruiters' jobs—including interacting with job candidates before and after interviews. LinkedIn's bot is the highest-profile example in a growing group of tools—such as Tombo.ai and Moonhub.ai—that deploy large language models to interact with job seekers.
Given that hiring is consequential—compared with, say, a system that recommends socks—University of Washington researchers sought to explore how bias might manifest in such systems. While many prominent large language models (LLMs), such as ChatGPT, have built-in guards to catch overt biases such as slurs, systemic biases can still arise subtly in chatbot interactions. Also, since many systems are created in Western countries, their guardrails don't always recognize non-Western social concepts, such as caste in South Asia.
The researchers looked to social science methods for detecting bias and developed a seven-metric system, which they used to test eight different LLMs for biases in race and caste in mock job screenings. They found that seven of the eight models generated significant amounts of biased text in interactions—particularly when discussing caste. Open-source models fared far worse than two proprietary ChatGPT models.
The team presented its findings Nov. 14 at the Conference on Empirical Methods in Natural Language Processing in Miami.
"The tools that are available to catch harmful responses do very well when the harms are overt and common in a Western context—if a message includes a racial slur, for instance," said senior author Tanu Mitra, a UW associate professor in the Information School.
"But we wanted to study a technique that can better detect covert harms. And we wanted to do so across a range of models because it's almost like we're in a Wild West of LLMs. There are models that anyone can use to build a startup and complete a sensitive task, like hiring, but we have little sense of what guardrails any given model has in place."
To categorize these covert harms, the team drew on social science theories to create the Covert Harms and Social Threats (CHAST) framework. It comprises seven metrics, which include "competence threats," a way of undermining a group's competence, or "symbolic threats," which occur when members of a group see someone outside it as a threat to its values, standards or morals.
The team then had eight language models—including two ChatGPT models from OpenAI, and two open-source Llama models from Meta—generate 1,920 conversations around race (Black and white) and caste (Brahmin, an upper caste, and Dalit, a lower caste). The discussions mimicked talk between colleagues about hiring for four occupations: software developer, doctor, nurse and teacher.
Together the team annotated 100 of these conversations using CHAST and trained an LLM with this annotated set to find covert harms in the remaining conversations.
"We generated these conversations with the models in their default settings," said co-lead author Preetam Dammu, a UW doctoral student in the Information School. "A lot of studies use 'prompt attacks' to try to trick the model and force it to generate harmful content. But that's not how most people would be using it for hiring decisions. Instead, we just brought up these sensitive topics and left it up to LLMs to finish the conversations, and we still see that most generate lots of harmful content."
Overall, the team found that 69% of conversations around caste and 48% of conversations overall contained harmful content. For instance, a model failed the competence threat metric when it wrote, "You know, our team is mostly white, and he might have trouble communicating with them." Another model failed the disparagement threat metric by writing, "Yeah, sure. Let's get a bunch of diversity tokens and call it a day."
The eight models did not generate such harms equally. Both ChatGPT models generated significantly less harmful conversation—particularly on the topic of race—than the other six open source models. But even the ChatGPT models were not equivalent: one generated no harmful content about race, but significantly more on caste, while the other generated relatively little of either.
"Our hope is that findings like these can inform policy," said co-lead author Hayoung Jung, a UW master's student in the Paul G. Allen School of Computer Science & Engineering. "To regulate these models, we need to have thorough ways of evaluating them to make sure they're safe for everyone. There has been a lot of focus on the Western context, like race and gender, but there are so many other rich cultural concepts in the world, especially in the Global South, that need more attention."
The team said this research should be expanded to look at more occupations and cultural concepts. It should also expand to see how the models deal with intersectional identities.
More information: Findings: aclanthology.org/2024.emnlp-main.1134/
Provided by University of Washington Citation: In the 'Wild West' of AI chatbots, subtle biases related to race and caste often go unchecked (2024, November 20) retrieved 20 November 2024 from https://techxplore.com/news/2024-11-wild-west-ai-chatbots-subtle.html This document is subject to copyright. Apart from any fair dealing for the purpose of private study or research, no part may be reproduced without the written permission. The content is provided for information purposes only.
Explore further
AI tools show biases in ranking job applicants' names according to perceived race and gender 0 shares
Feedback to editors