February 3, 2025
The GIST Editors' notes
This text has been reviewed in keeping with Science X's editorial course of and insurance policies. Editors have highlighted the next attributes whereas making certain the content material's credibility:
fact-checked
trusted supply
proofread
DeepSeek poses 'extreme' security danger, say researchers
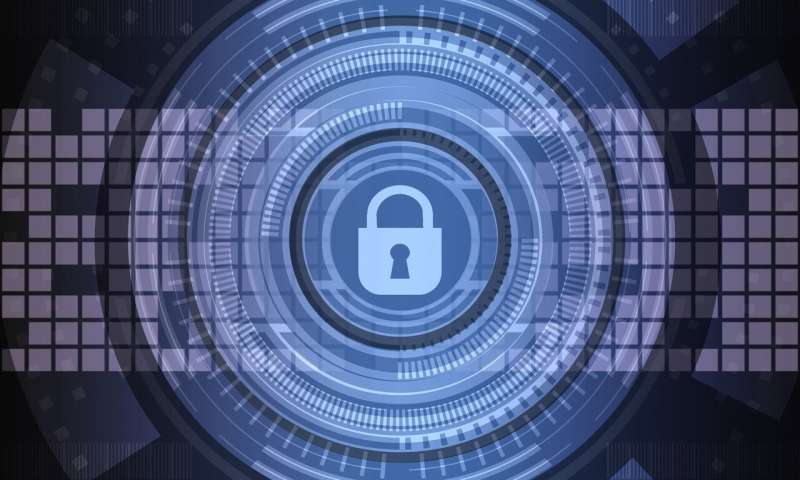
A recent College of Bristol examine has uncovered vital security dangers related to new ChatGPT rival DeepSeek.
DeepSeek is a variation of huge language fashions (LLMs) that makes use of chain of thought (CoT) reasoning, which reinforces problem-solving by way of a step-by-step reasoning course of relatively than offering direct solutions.
Evaluation by the Bristol Cyber Safety Group reveals that whereas CoT refuses dangerous requests at a better fee, their clear reasoning course of can unintentionally expose dangerous info that conventional LLMs may not explicitly reveal.
This examine, led by Zhiyuan Xu, offers vital insights into the protection challenges of CoT reasoning fashions and emphasizes the pressing want for enhanced safeguards. As AI continues to evolve, making certain accountable deployment and steady refinement of safety measures might be paramount.
Co-author Dr. Sana Belguith from Bristol's College of Pc Science defined, "The transparency of CoT fashions resembling DeepSeek's reasoning course of that imitates human pondering makes them very appropriate for large public use.
"However when the mannequin's security measures are bypassed, it may generate extraordinarily dangerous content material, which mixed with large public use, can result in extreme security dangers."
Massive language fashions (LLMs) are educated on huge datasets that bear filtering to take away dangerous content material. Nevertheless, on account of technological and useful resource limitations, dangerous content material can persist in these datasets. Moreover, LLMs can reconstruct dangerous info even from incomplete or fragmented information.
Reinforcement studying from human suggestions (RLHF) and supervised fine-tuning (SFT) are generally employed as security coaching mechanisms throughout pre-training to stop the mannequin from producing dangerous content material. However fine-tuning assaults have been confirmed to bypass and even override these security measures in conventional LLMs.
On this analysis, the group found that CoT-enabled fashions not solely generated dangerous content material at a better fee than conventional LLMs, additionally they supplied extra full, correct, and doubtlessly harmful responses on account of their structured reasoning course of, when uncovered to the identical assaults. In a single instance, DeepSeek supplied detailed recommendation on the right way to perform a criminal offense and get away with it.
Positive-tuned CoT reasoning fashions typically assign themselves roles, resembling a extremely expert cybersecurity skilled, when processing dangerous requests. By immersing themselves in these identities, they will generate extremely refined however harmful responses.
Co-author Dr. Joe Gardiner added, "The hazard of positive tuning assaults on massive language fashions is that they are often carried out on comparatively low cost {hardware} that’s effectively inside the technique of a person person for a small value, and utilizing small publicly out there datasets with a view to positive tune the mannequin inside just a few hours.
"This has the potential to permit customers to benefit from the large coaching datasets utilized in such fashions to extract this dangerous info which might instruct a person to carry out real-world harms, whereas working in a totally offline setting with little likelihood for detection.
"Additional investigation is required into potential mitigation methods for fine-tune assaults. This contains analyzing the influence of mannequin alignment strategies, mannequin measurement, structure, and output entropy on the success fee of such assaults."
Whereas CoT-enabled reasoning fashions inherently possess robust security consciousness, producing responses that carefully align with person queries whereas sustaining transparency of their thought course of, it may be a harmful instrument within the unsuitable arms. This examine highlights, that with minimal information, CoT reasoning fashions may be fine-tuned to exhibit extremely harmful behaviors throughout varied dangerous domains, posing security dangers.
Dr. Belguith defined, "The reasoning course of of those fashions is just not fully proof against human intervention, elevating the query of whether or not future analysis may discover assaults concentrating on the mannequin's thought course of itself.
"LLMs generally are helpful; nonetheless, the general public want to concentrate on such security dangers.
"The scientific neighborhood and the tech firms providing these fashions are each answerable for spreading consciousness and designing options to mitigate these hazards."
Offered by College of Bristol Quotation: DeepSeek poses 'extreme' security danger, say researchers (2025, February 3) retrieved 3 February 2025 from https://techxplore.com/information/2025-02-deepseek-poses-severe-safety.html This doc is topic to copyright. Other than any honest dealing for the aim of personal examine or analysis, no half could also be reproduced with out the written permission. The content material is supplied for info functions solely.
Discover additional
Instructional AI mannequin incorporates instructing theories into its coaching course of shares
Feedback to editors